The Role of Data Collection Machine Learning in Advancing Artificial Intelligence
- shalinigts16
- Feb 12, 2024
- 4 min read
I. Introduction
Artificial Intelligence (AI) is rapidly reshaping the landscape of various industries, driving innovations and efficiencies in areas as diverse as healthcare, automotive, finance, and customer service. At its core, AI involves the creation of systems that can perform tasks which traditionally require human intelligence. This includes problem-solving, recognizing patterns, and understanding language.
Machine Learning (ML), a crucial subset of AI, is particularly focused on developing algorithms that enable computers to learn and make decisions based on data. Unlike traditional software, where human programmers define all decisions and actions, machine learning algorithms adjust their behavior based on the data they process, allowing them to make predictions or decisions without being explicitly programmed for each contingency.
The effectiveness of these AI and ML systems is heavily reliant on the data they are trained with. Data collection, the process of gathering information from various sources to build datasets, is a critical step in this process. It's the quality, diversity, and volume of this collected data that largely determine the success of AI applications. This blog post will explore the intricate role of data collection in machine learning and how it propels the advancement of AI.

II. Understanding Data Collection in Machine Learning
In machine learning, data collection is the foundation upon which models are built. It involves accumulating information that can be used to train, test, and improve algorithms. Data in ML can be categorised into three types:
Structured Data: This is highly organised and easily searchable data, often stored in databases. Examples include Excel files or SQL databases, where the data is organised into rows and columns.
Unstructured Data: This type of data is unorganised and includes things like text, images, and videos. It's more complex to process and analyse but is critical for many advanced AI applications like natural language processing and computer vision.
Semi-Structured Data: Falling between structured and unstructured, this data type includes elements of both, like JSON or XML files.
The tools and methods for data collection in AI are varied. They range from automated web scraping tools and APIs to collect online data, to using IoT sensors for real-time data collection in physical environments. The choice of tools often depends on the data needs of the specific ML application.
III. The Impact of Quality Data on Machine Learning Models
The accuracy and reliability of a machine learning model are directly tied to the quality of the data used for training. High-quality data must be representative, unbiased, and comprehensive. It should accurately reflect the real-world scenario the model is intended to solve. For instance, in facial recognition technology, a diverse dataset representing various ethnicities, ages, and lighting conditions is crucial for the model’s accuracy and fairness.
However, collecting such high-quality data can be challenging. Issues like selection bias, where the data collected is not representative of the broader population, or data contamination, where incorrect or irrelevant data is introduced, can severely impact model performance. Addressing these challenges often involves implementing robust data validation and cleaning processes, as well as adopting strategies to ensure data diversity and representation.
IV. Ethical and Privacy Concerns in Data Collection
Data collection raises significant ethical and privacy concerns. The process must respect individual privacy and comply with legal standards. Key considerations include obtaining consent from individuals whose data is being collected, ensuring data security, and maintaining transparency about how the data will be used.
Regulations like the General Data Protection Regulation (GDPR) in the European Union have set standards for data collection practices, emphasising the importance of user consent and data protection. Companies and researchers must navigate these regulations carefully, balancing the need for comprehensive data with ethical considerations and privacy laws.
V. Future Trends and Innovations in Data Collection for AI
The future of data collection in AI is poised for significant advancements thanks to emerging technologies. The Internet of Things (IoT), for instance, is enabling the collection of vast amounts of real-time data from sensors embedded in various devices. This data is invaluable for training more responsive and context-aware AI models.
Big data analytics is another area transforming data collection. With the ability to process and analyse large datasets, AI systems can uncover patterns and insights that were previously inaccessible. Additionally, edge computing, where data processing occurs closer to where data is collected, is set to reduce latency and improve the efficiency of AI systems.
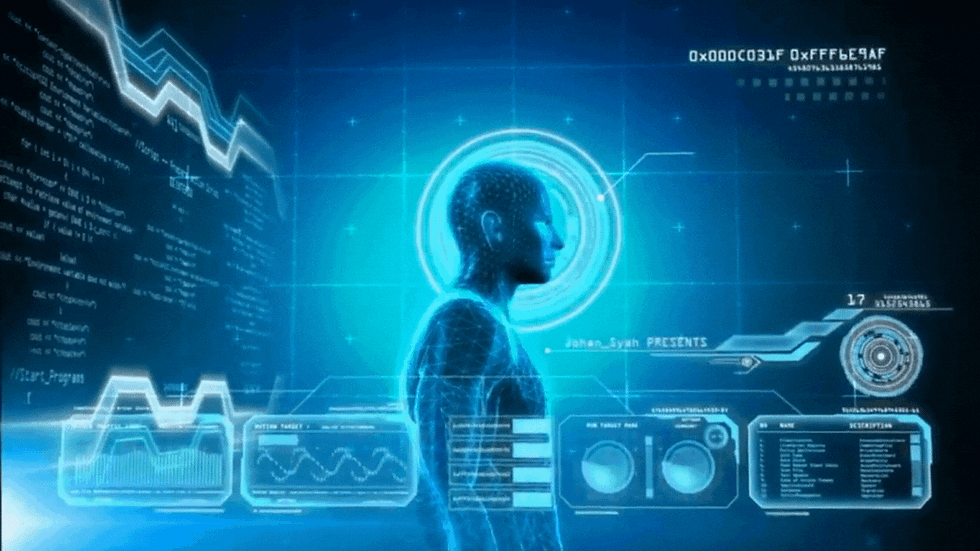
VI. Conclusion
In conclusion, data collection is a fundamental pillar in the field of AI and machine learning. The journey of AI from a concept to a transformative technology is underpinned by the effectiveness of data collection strategies. As we advance, it's vital for professionals in this field to not only focus on collecting vast amounts of data but also to pay heed to the quality, ethical considerations, and privacy implications of their data collection practices.
Enhancing AI Projects with Globose Technology Solutions: Expert Data Collection and Annotation Services
The Globose Technology Solutions (GTS) specialises in AI data collection services, providing diverse datasets such as images, videos, speech, and text to train machine learning models. Their services cover data annotation, transcription, and collection across various industries, including technology, financial services, retail, healthcare, automotive, and government sectors. They emphasise quality assurance, extensive expertise in micro-tasks, and a large, globally distributed workforce. GTS's offerings are designed to enhance AI projects through meticulous data labelling, streamlined data operations, and efficient production pipelines. This comprehensive approach can significantly benefit clients seeking specialised, high-quality datasets for their AI and machine learning endeavours.
Comments