Faces of Tomorrow: A Compact Face Image Dataset for Predictive Analysis
- shalinigts16
- Mar 15, 2024
- 3 min read
Introduction
In the realms of machine learning and computer vision, face image datasets are indispensable. They provide the raw material for algorithms to learn patterns and make predictions about human faces, from identifying individuals to guessing their emotions. However, as technology advances, the need for compact face image datasets has become increasingly evident. These datasets, with their efficient storage and faster processing capabilities, are becoming the backbone of modern computational systems.

The Need for Compact Face Image Datasets
Large face image datasets, while rich in information, come with their own set of challenges. They demand substantial storage space and consume considerable computational resources, making them less practical for real-time applications. Compact face image datasets address these issues by minimising redundancy and preserving only the most relevant features. This reduction not only alleviates storage concerns but also accelerates processing speeds, enabling quicker and more efficient analyses.
Overview of "Faces of Tomorrow"
Faces of Tomorrow is a compact face image dataset designed for predictive analysis. It comprises a curated collection of face images, carefully compressed and formatted to maintain essential characteristics while reducing overall size. The dataset utilises advanced image compression techniques and feature selection methods to ensure that each image retains its predictive utility despite the reduction in size.
Applications in Predictive Analysis
The Faces of Tomorrow face image dataset finds its applications in various predictive analysis tasks. It is instrumental in facial recognition systems, where it helps in identifying individuals quickly and accurately. In age estimation, the dataset aids in predicting a person's age based on facial features. Similarly, it is used in emotion detection algorithms to interpret human emotions from facial expressions. These applications demonstrate the versatility and effectiveness of compact face image datasets in predictive analysis.
Challenges and Limitations
However, using compact face image datasets is not without challenges. The process of reducing dataset size can lead to a loss of information, which might affect the accuracy of predictions in certain scenarios. Additionally, compact datasets may not capture the full variability of real-world data, limiting their applicability. To mitigate these issues, researchers employ advanced algorithms and image annotation services to enhance the dataset's representativeness and reliability.
Enhancing Predictive Analysis with Compact Face Image Datasets
The advancement of machine learning algorithms has led to remarkable progress in facial recognition technology. However, the efficacy of these algorithms heavily relies on the quality and efficiency of the underlying face image datasets. Compact face image datasets, such as "Faces of Tomorrow," are at the forefront of this evolution, offering a streamlined approach to data handling and processing.
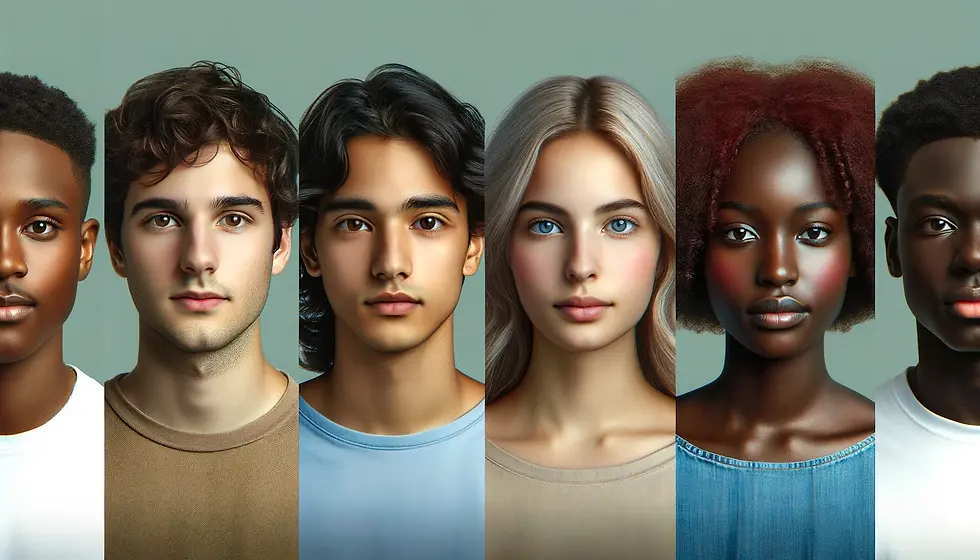
Future Directions
The future of compact face image datasets looks promising, with potential advancements in compression techniques and new applications in emerging fields like augmented reality (AR) and virtual reality (VR). As technology evolves, these datasets will play a crucial role in developing more sophisticated and efficient predictive systems. Continued research and development in this area are essential to unlock the full potential of compact face image datasets.
Innovative Techniques for Dataset Compression
Creating a compact face image dataset involves employing innovative compression techniques that reduce file size without compromising the integrity of the data. Techniques such as principal component analysis (PCA) and deep learning-based autoencoders are commonly used to extract and retain essential features while discarding redundant information. This approach ensures that the dataset remains robust and effective for predictive analysis.
Conclusion
In conclusion, Faces of Tomorrow exemplifies the significance of compact “face image datasets” in the field of predictive analysis. By enabling efficient storage and processing, these datasets pave the way for faster and more accurate predictions. As we move forward, their role in driving innovation across various applications will undoubtedly continue to grow.
How GTS.AI Elevates Data Collection for Machine Learning Initiatives
At Globose Technology Solutions Pvt Ltd, we excel in providing "Face Image Dataset" services for your machine learning projects. Our expertise in data collection, paired with our cutting-edge technology, ensures that we deliver high-quality, relevant face image datasets to train your machine learning models effectively. Our team is adept at overcoming common challenges associated with face image datasets, such as dealing with imbalanced data and addressing missing or incomplete images. We utilise advanced techniques to ensure that your face image datasets are balanced, accurate, and comprehensive.
Comentarios