Building an Invoice Dataset Collection: Challenges and Best Practices
- shalinigts16
- Sep 29, 2023
- 3 min read
Introduction:
In the modern business landscape, the digitization of invoices has become a crucial aspect of streamlining financial processes and enhancing operational efficiency. As Artificial Intelligence (AI) and Machine Learning (ML) continue to revolutionize various industries, training robust Invoice dataset collection is a Processing system that requires a high-quality dataset. Collecting an effective and diverse dataset is a challenging task, but it forms the backbone of building accurate and reliable ML models.
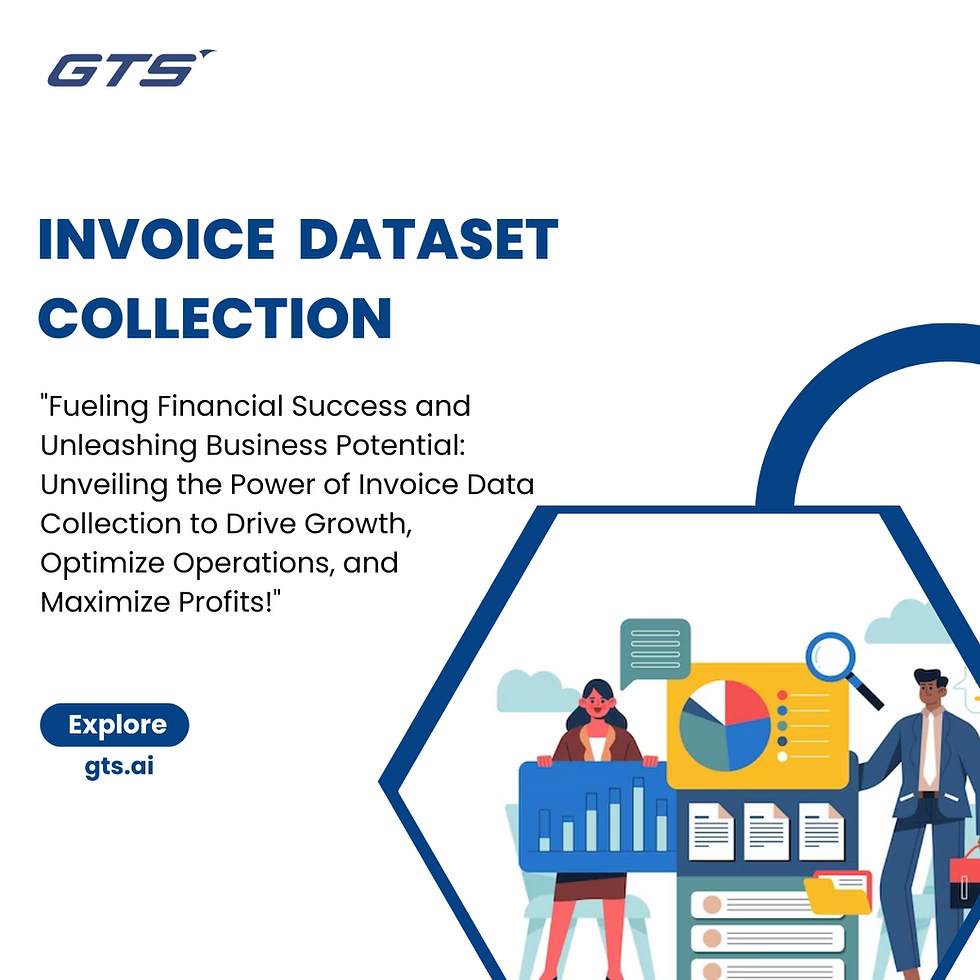
Challenges in Invoice Dataset Collection:
Data Availability and Accessibility: One of the primary challenges in building an invoice dataset is obtaining a sufficient quantity of diverse and representative invoices. Companies often face hurdles in accessing invoice data due to privacy concerns, data ownership, or contractual limitations. Additionally, historical paper-based invoices may not be readily available in a digital format, making the collection process more tedious.
Data Anonymization and Privacy: Invoice data often contains sensitive information, including customer details, financial transactions, and pricing. Ensuring data privacy and anonymity is paramount when collecting invoice datasets, especially if the data originates from multiple sources or external partners.
Data Labelling and Annotation: Accurate data labeling is essential for supervised ML models. In the context of invoice data, this involves annotating key information such as invoice numbers, dates, line items, and totals. Manual data labeling can be time-consuming and error-prone, necessitating efficient annotation techniques and quality control processes.
Data Imbalance: In real-world scenarios, the distribution of invoice types may be uneven, leading to data imbalance in the dataset. A disproportionate representation of certain invoice layouts or formats can impact the ML model's performance and lead to biased results.
Variability in Invoice Formats: Different companies and industries use diverse invoice formats, layouts, and languages. ML models must be trained on a dataset that accounts for this variability to ensure the system's adaptability to new or previously unseen invoice types.
Best Practices for Invoice Dataset Collection:
Collaborate with Partners and Clients: Establish partnerships with clients, vendors, and other stakeholders to request permission for data sharing and collaborate on dataset creation. This collaborative effort can ensure access to diverse and real-world invoice samples.
Data Anonymization and Compliance: Prioritise data privacy and compliance with regulations like GDPR when collecting and sharing invoice data. Anonymize sensitive information to safeguard the privacy of individuals and businesses involved.
Data Augmentation: To overcome data scarcity or imbalances, consider data augmentation techniques such as rotation, flipping, and synthetic data generation. These methods can increase the diversity and size of the dataset, enhancing model performance.
Semi-Automated Annotation: Use semi-automated annotation tools that combine human expertise with AI capabilities to expedite the data labelling process while maintaining accuracy.
Quality Control and Validation: Implement stringent quality control measures to verify the accuracy and consistency of data annotations. Regularly validate the dataset to identify and rectify any inconsistencies or errors.
Continuous Update and Expansion: Invoice formats and layouts can evolve over time. Ensure the dataset remains up-to-date by periodically incorporating new samples and expanding its scope to accommodate emerging trends.
Conclusion:
Building an effective invoice dataset collection is fundamental in developing robust AI-powered Invoice Processing systems. The challenges involved in obtaining diverse, accurately labeled, and privacy-compliant data are significant. However, by embracing best practices like collaboration, data anonymization, augmentation, and continuous updates, companies can create a dataset that empowers their ML models to accurately interpret and process invoices from various sources. A well-curated and comprehensive dataset is the key to unlocking the full potential of AI-driven invoice processing, enabling businesses to streamline financial operations and drive growth in today's competitive landscape.
At Globose Technology Solutions Pvt Ltd (GTS), we understand the significance of selecting the right data collection company for your machine learning endeavors. With our expertise in data collection, curation, and quality assurance, we provide tailored solutions that drive the success of your projects. Contact us today to discuss how our focus on data collection can empower your machine-learning initiatives and propel your business forward.
Comments