Introduction:
Optical Character Recognition (OCR) is a game-changing technology that allows computers to interpret and convert various types of documents, images, and handwritten text into editable and machine-readable formats. OCR has revolutionised data extraction, document digitization, and information retrieval processes across industries. To build accurate and robust OCR models, access to high-quality training data is crucial. In this blog, we present a comprehensive list of OCR datasets that are invaluable resources for training OCR machine learning models.
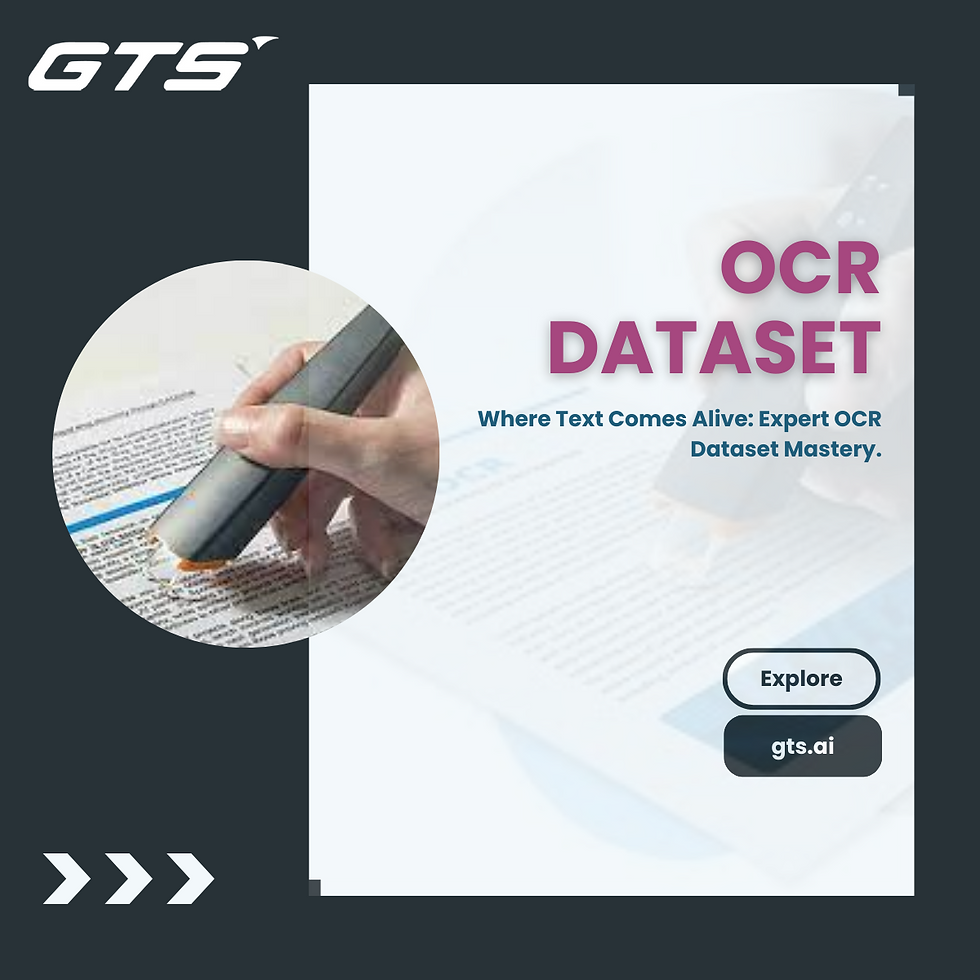
MNIST (Modified National Institute of Standards and Technology):The MNIST dataset is one of the most widely used benchmarks in OCR research. It consists of 28x28 grayscale images of handwritten digits (0 to 9) and their corresponding labels. While primarily used for digit recognition, MNIST serves as an excellent starting point for OCR beginners due to its simplicity and accessibility.
IAM Handwriting Database:This dataset focuses on handwritten English text recognition. It contains more complex and varied text samples compared to MNIST. The IAM Handwriting Database includes text lines written by different individuals, allowing OCR models to learn diverse handwriting styles and variations.
Street View Text (SVT) Dataset:The SVT dataset is designed for scene text recognition, simulating real-world scenarios where text is captured in natural environments like street signs or storefronts. The dataset contains images of scene text along with corresponding annotations, providing a challenging and practical OCR training resource.
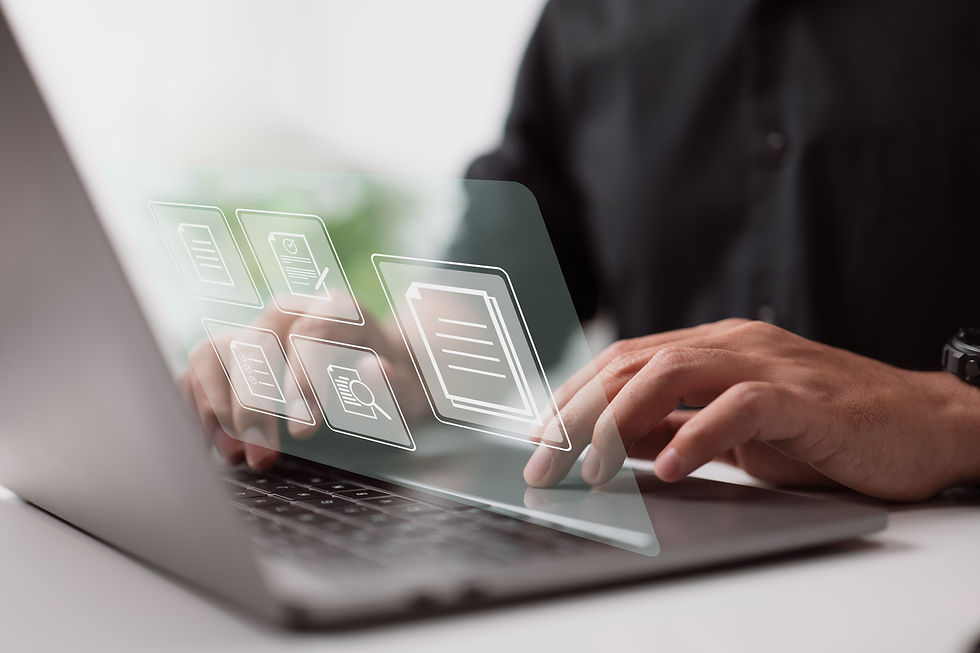
IIIT 5K-Words Dataset:Similar to SVT, the IIIT 5K-Words Dataset focuses on scene text recognition. It consists of images collected from the web, capturing text in various languages and fonts. This dataset offers a broader scope for OCR models to handle multilingual and diverse textual content.
CORD Dataset:The CORD dataset caters to OCR needs in the medical domain. It comprises a collection of scientific papers related to COVID-19, enabling the training of OCR models to extract valuable information from research documents.
CAPTCHA Images:CAPTCHA images, designed to prevent automated bots from accessing websites, can serve as interesting OCR training data. Though challenging due to image distortions and obfuscations, using CAPTCHA images can help OCR models improve their robustness and accuracy.
Tobacco3482:The Tobacco3482 dataset is specifically tailored for OCR in historical documents. It contains images of tobacco advertisements from the early 20th century, offering unique challenges in recognizing older fonts and styles.
UNLV-ISRI-ALPR Dataset: This dataset focuses on Automatic License Plate Recognition (ALPR). It includes images of license plates with annotations, enabling OCR models to recognize alphanumeric characters present on license plates accurately.
Conclusion:
As a leading technology solution provider, Globose Technology Solutions Pvt Ltd (GTS) recognizes that OCR datasets are the bedrock of successful OCR models. These datasets empower researchers and practitioners to push the boundaries of text recognition technology. With our commitment to cutting-edge solutions and a dedication to advancing OCR research, GTS stands as your partner in harnessing the power of OCR datasets for building accurate and innovative OCR solutions.
コメント