Introduction:
Optical Character Recognition (OCR) is a revolutionary technology that enables machines to interpret printed or handwritten text from images or scanned documents. This powerful capability finds applications in various industries, including document digitization, text extraction, and data analysis. To develop accurate and robust OCR models, the foundation lies in the quality and diversity of the training data. In this blog, we will explore the top OCR training datasets that serve as the building blocks for creating high-performing text recognition models.
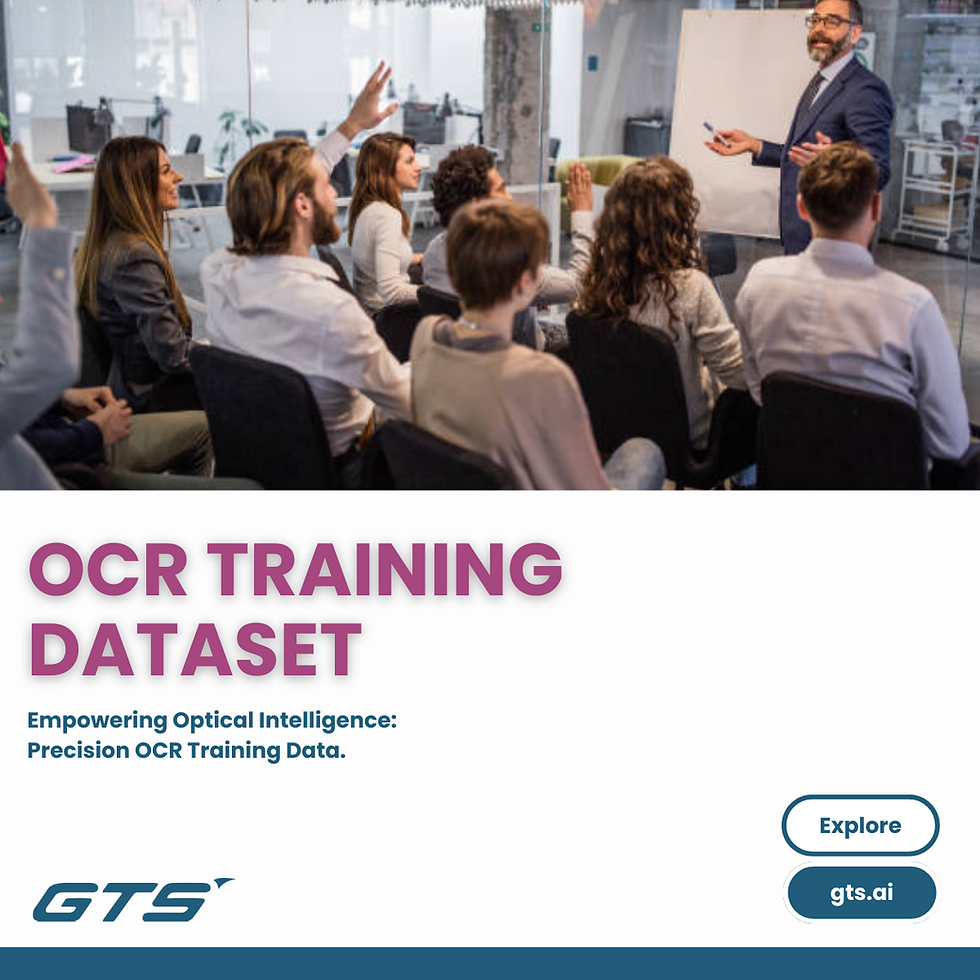
The Significance of OCR Training Datasets:
OCR training datasets act as the bedrock for teaching machine learning algorithms how to recognize and understand different characters, fonts, and languages. The more comprehensive and diverse the dataset, the better the OCR model's ability to handle variations in text, layouts, and writing styles. A well-curated dataset can significantly enhance the accuracy and generalisation capabilities of OCR models, making them indispensable for real-world applications.
MNIST Dataset: The MNIST dataset is one of the most popular and widely used datasets for OCR training, especially for handwritten digit recognition. It consists of 28x28 grayscale images of handwritten digits from 0 to 9. While originally intended for digit recognition, it has also been extended for character recognition tasks, making it a valuable resource for building basic OCR models.
IAM Handwriting Database: For projects requiring handwritten text recognition, the IAM Handwriting Database is an excellent choice. It contains over 13,000 isolated and labelled handwritten text lines, encompassing various writing styles and complexities. This dataset enables OCR models to learn the nuances of different handwriting styles and enhances their adaptability to real-world scenarios.
Tesseract Training Data: Tesseract is one of the most popular open-source OCR engines, developed by Google. It comes with its own training data, which can be used to fine-tune the OCR model for specific tasks. Tesseract training data includes various language packs, allowing users to train models for different languages and character sets.

SynthText: SynthText is a unique dataset designed to enhance the OCR model's ability to recognize text in natural scenes. It contains over 800,000 images with synthetic text superimposed on diverse backgrounds. The dataset helps OCR models become more robust in handling challenges posed by real-world scenarios, such as complex backgrounds, different lighting conditions, and varying text orientations.
ICDAR Robust Reading Competitions Datasets: The International Conference on Document Analysis and Recognition (ICDAR) hosts robust reading competitions, producing datasets for OCR model evaluation. These datasets comprise images captured in challenging conditions, such as low resolution, blurred text, and distorted fonts. Leveraging these datasets for training can significantly improve an OCR model's resilience to adverse conditions.
Conclusion:
As a leading technology solutions provider, Globose Technology Solutions Pvt Ltd (GTS) recognizes the integral role of OCR training datasets in the development of accurate and reliable text recognition models. These datasets lay the groundwork for the advancement of OCR technology. With GTS's dedication to innovation and excellence, we stand as your partner in harnessing the power of top-tier OCR training datasets to create state-of-the-art OCR solutions.
Comments