Introduction:
OCR training datasets play a crucial role in improving the accuracy and performance of OCR systems. These datasets consist of annotated images or documents that are used to train machine learning models to recognize and extract text from various sources.
Importance of High-Quality OCR Training Datasets
OCR training datasets serve as the foundation for developing accurate and robust OCR models. Here, we will delve into the significance of using high-quality training datasets for achieving superior OCR performance. We will discuss how the diversity, quantity, and accuracy of the data impact the training process and subsequent recognition accuracy.
Challenges in Creating OCR Training Datasets
Creating OCR training datasets poses several challenges due to the complex nature of text in real-world scenarios. In this section, we will explore the hurdles faced in collecting and annotating training data. We will discuss issues related to data acquisition, data labeling, and ensuring the dataset's representativeness to handle diverse text styles, languages, fonts, and document layouts.
Conclusion:
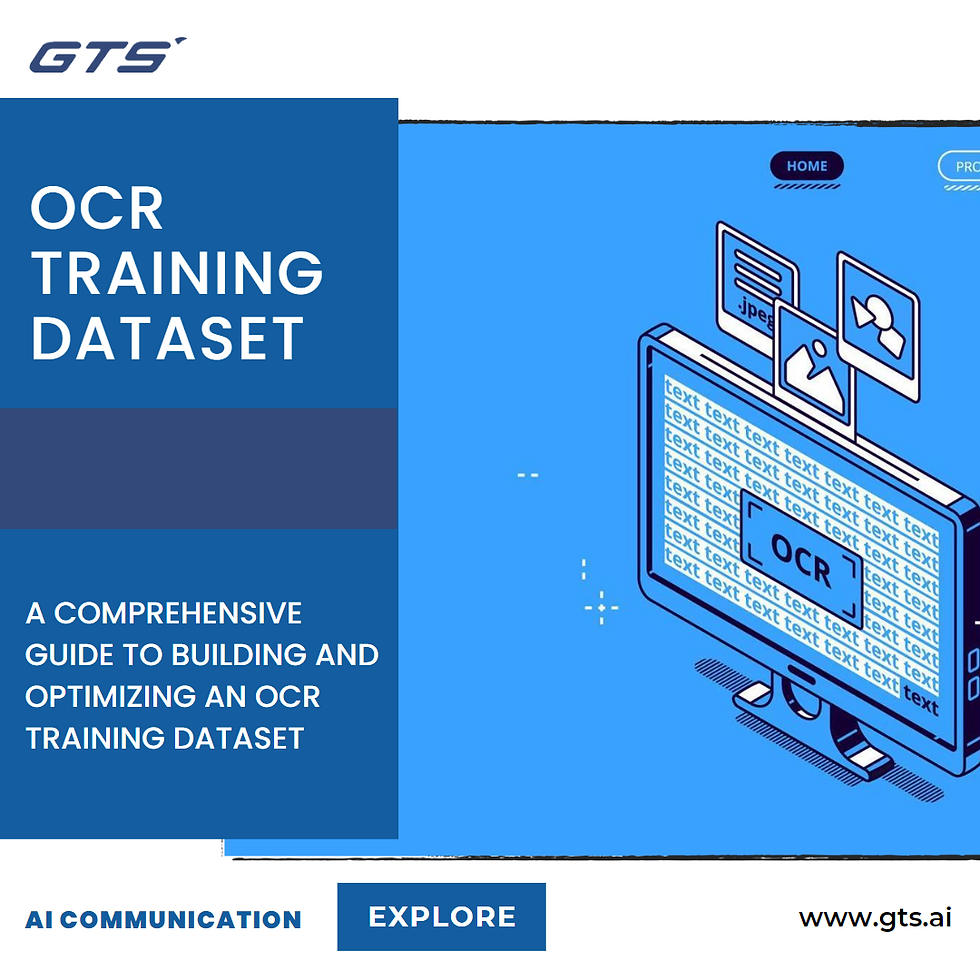
In conclusion, the training dataset for Optical Character Recognition (OCR) plays a critical role in the accuracy and performance of OCR systems. OCR is a technology that converts printed or handwritten text into machine-readable text, enabling computers to interpret and analyze the content.
The training dataset for OCR typically consists of a large collection of images or scanned documents that contain a variety of text samples in different fonts, sizes, styles, and languages. These images are paired with their corresponding ground truth or reference text, which represents the correct text that should be recognized from each image.
Σχόλια